Diagnosing Alzheimer’s Using AI
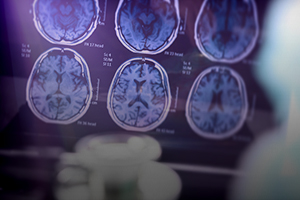
In another application of machine learning, researchers have been working on an algorithm to predict the likelihood of Alzheimer’s disease (AD) using positron emission tomography (PET) scans. PET measures the uptake of radioactive compounds by brain cells to detect subtle changes in metabolism. One parameter that is easily measured by this technique is glucose consumption – an early predictor of AD.
The algorithm was “trained” using images from a 12-year study of patients 55 years of age and older, some of whom were ultimately diagnosed with AD or milder forms of dementia and some with no memory declines. Over 2,000 PET scans from about 1,000 subjects were included. Approximately 90 percent of the scans were used to train the system. It was then tested on the other 10 percent, and results showed that it correctly predicted AD 81 percent of the time. It was then used to test an additional independent data set taken from 40 patients over a 10-year period. The algorithm correctly identified 100 percent of those patients who would be diagnosed with AD an average of six years later.
Further work will focus on catching the warning signs even earlier in the development of the disease, when therapies are most effective.
For information: Jae Ho Sohn, M.D., University of California-San Francisco Medical Center, Department of Radiology, 550 Parnassus Avenue, San Francisco, CA 94143; email: sohn87@gmail.com; Web site: https://www.ucsf.edu/ or https://www.ucsf.edu/topics/alzheimers-and-dementia